 |
Personen > Joachim Funke > CPS Review
Complex problem solving research in North America and Europe: An integrative review
Joachim Funke
Department of Psychology, University of Heidelberg, Germany
Peter Frensch
Department of Psychology,
Humboldt University, Berlin, Germany
© 1995 by Joachim Funke & Peter Frensch
Abstract
Complex problem solving is a relatively new
research domain that is based on the assumption that complex,
real-life problem solving has been largely ignored by traditional
problem solving research. In this article, we contrast the two
dominant approaches to studying complex problem solving, the North
American and the European approaches. We present a definition
of complex problem solving and describe a theoretical framework
that accommodates the theoretical and empirical strides that have
been made in understanding complex problem solving thus far, and
may serve as a guide for future research. We discuss the dominant
methodological approaches that have been employed to study complex
problem solving, and offer our own recommendations on which of
the various approaches might be the most promising one.
Introduction
Many of our daily activities involve complex
problem solving (henceforth CPS) of some sort. For example, we
decide how to structure a theoretical paper on an academic topic,
how to fix a car that has broken down, make plans for an extended
vacation, and so on. Of course, not all problem solving is alike.
There are problems that can be solved with relatively few mental
steps, and there are problems that require extensive "thinking.
There are problems that we have never encountered before, and
there are problems we are familiar with. There are problems that
have very clear goals, and there are problems where the goals
are far from clear. Complex problems, then, can be distinguished
on any number of meaningful dimensions, and the solution processes,
the mental steps we engage in when solving a problem, may differ
widely for different types of problems.
In this article, we summarize the present dominant empirical approaches
to studying complex problem solving, after providing a brief historical
background within which the development of the dominant approaches
in North America and Europe can be understood. In addition, we
present a definition of complex problem solving, and describe
a theoretical framework that accommodates the theoretical and
empirical strides that have been made in understanding complex
problem solving thus far, and serves as a guide for future research.
Last but not least, we discuss the dominant methodological approaches
that have been employed to study CPS, and offer our own recommendations
on which approach might be the most promising.
Historical Roots and Current
Situation
Beginning with the early experimental work
of the Gestaltists in Germany (e.g., Duncker, 1935), and continuing
through the sixties and early seventies, research on problem solving
was typically conducted with relatively simple, laboratory tasks
(e.g., Duncker's "X-ray problem; Ewert & Lambert's,
1932, "disk problem, later known as "Tower of
Hanoi) that were novel to subjects (e.g., Mayer, 1992).
Simple novel tasks were used for various reasons: they had clearly
defined optimal solutions, they were solvable within a relatively
short time frame, subjects' problem solving steps could be traced,
and so on. The underlying assumption was, of course, that simple
tasks, such as the "Tower of Hanoi, captured the main
properties of "real problems, and that the cognitive
processes underlying subjects' solution attempts on simple problems
were representative of the processes engaged in when solving "real
problems. Thus, simple problems were used for reasons of convenience,
and generalizations to more complex problems were thought possible.
Perhaps the best known and most impressive example of this line
of research is the work by Newell and Simon (1972).
However, beginning in the seventies, researchers became increasingly
convinced that empirical findings and theoretical concepts derived
from simple laboratory tasks were not generalizable to more complex,
real-life problems. Even worse, it appeared that the processes
underlying CPS in different domains were different from each other
(Sternberg, 1995). These realizations have led to rather different
responses in North America and Europe.
In North America, initiated by the work of Herbert Simon on learning
by doing in semantically rich domains (e.g., Anzai & Simon,
1979; Bhaskar & Simon, 1977), researchers began to investigate
problem solving separately in different natural knowledge domains
(e.g., physics, writing, chess playing) thus relinquishing on
their attempts to extract a global theory of problem solving (e.g.,
Sternberg & Frensch, 1991). Instead, these researchers have
frequently focused on the development of problem solving within
a certain domain, that is on the development of expertise (e.g.,
Anderson, Boyle, & Reiser, 1985; Chase & Simon, 1973;
Chi, Feltovich, & Glaser, 1981). Areas that have attracted
rather intensive attention in North America include such diverse
fields as reading (Stanovich & Cunningham, 1991), writing
(Bryson, Bereiter, Scardamalia, & Joram, 1991), calculation
(Sokol & McCloskey, 1991), political decision making (Voss,
Wolfe, Lawrence, & Engle, 1991), managerial problem solving
(Wagner, 1991), lawyers' reasoning (Amsel, Langer, & Loutzenhiser,
1991), mechanical problem solving (Hegarty, 1991), problem solving
in electronics (Lesgold & Lajoie, 1991), computer skills (Kay,
1991), game playing (Frensch & Sternberg, 1991), and personal
problem solving (Heppner & Krauskopf, 1987).
In Europe, two main approaches have surfaced, one initiated by
Donald Broadbent (1977; see Berry & Broadbent, 1995) in Great
Britain and the other one by Dietrich Dörner (1975, 1985;
see Dörner & Wearing, 1995) in Germany. The two approaches
have in common an emphasis on relatively complex, semantically
rich, computerized laboratory tasks that are constructed to be
similar to real-life problems. The approaches differ somewhat
in their theoretical goals and methodology, however. The tradition
initiated by Broadbent emphasizes the distinction between cognitive
problem solving processes that operate under awareness versus
outside of awareness, and typically employs mathematically well-defined
computerized systems. The tradition initiated by Dörner,
on the other hand, is interested in the interplay of the cognitive,
motivational, and social components of problem solving, and utilizes
very complex computerized scenarios that contain up to 2,000 highly
interconnected variables (e.g., Dörner, Kreuzig, Reither,
& Stäudel's, 1983, LOHHAUSEN project; Ringelband, Misiak,
& Kluwe, 1990). The two traditions are described in detail
by Buchner (1995).
To sum up, researchers' realization that problem solving processes
differ across knowledge domains and across levels of expertise
(e.g., Sternberg, 1995) and that, consequently, findings obtained
in the laboratory cannot necessarily be generalized to problem
solving situations outside the laboratory, has during the past
two decades, led to an emphasis on real-world problem solving.
This emphasis has been expressed quite differently in North America
and Europe, however. Whereas North American research has typically
concentrated on studying problem solving in separate, natural
knowledge domains, much of the European research has focused on
novel, complex problems, and has been performed with computerized
scenarios (see Funke, 1991, for an overview).
Complex Problem Solving: A Definition
With the above discussion in mind, it might
not come as a surprise to find that there exists a wide variety
of definitions of the term complex problem solving that
all have little in common (e.g., Frensch & Funke, 1995).
Indeed, researchers in the area of problem solving have long been
troubled by the absence of agreement on the exact meaning of many
of the basic terms in the area (e.g., Smith, 1991). Any general
conclusion regarding complex problem solving, however, and any
theoretical model of complex problem solving can only be meaningful
if we can all agree on what constitutes a problem and what constitutes
complex problem solving. Below we therefore offer our own definition
of CPS, a definition that is firmly rooted in the European tradition
of Donald Broadbent and Dietrich Dörner. The definition
has the advantage that it incorporates many aspects of the definitions
provided by the contributors to the volume edited by Frensch and Funke (1995), and thus is rooted in a rather broad theoretical basis. According to our definition,
CPS occurs to overcome barriers between a given state and a desired
goal state by means of behavioral and/or cognitive, multi-step
activities. The given state, goal state, and barriers between
given state and goal state are complex, change dynamically during
problem solving, and are intransparent. The exact properties
of the given state, goal state, and barriers are unknown to the
solver at the outset. CPS implies the efficient interaction between
a solver and the situational requirements of the task, and involves
a solver's cognitive, emotional, personal, and social abilities
and knowledge.
Notice that this definition differs rather
substantially from definitions that feature prominent in the North
American tradition. John Anderson, for the North American approach,
for example, has defined problem solving as "any goal-directed
sequence of cognitive operations (Anderson, 1980, p. 257),
regardless of whether the task is novel or familiar to the solver,
regardless of whether or not the task is complex, and regardless
of whether or not a single barrier or multiple barriers exist
between given state and goal state. Our definition, in contrast,
constrains potential problems by requiring that they be (a) novel
tasks that subjects are unfamiliar with, (b) complex, (c) dynamically
changing over time, and (d) intransparent. In order to solve
these problems, a solver has to be able to anticipate what will
happen over time, and has to consider side effects of potential
actions.
Note that the definition given above has an
important component, namely a focus on the distance between
the task and the solver, rather than a focus on the nature of
the task itself. That is, a complex problem is said to exist
only if there is a "gap between task and solver, or
a "barrier between the state given in the actual situation
and the goal state in the head of the problem solver. Therefore,
our definition can be called a "gap" definition. A
complex problem is not defined by task features, but rather by
the interaction between task requirements and solver, that is,
by the interaction between task characteristics and person characteristics.
Gap definitions, in general, imply that the same task may constitute
a problem for one solver, but not for another, whereas "task
oriented definitions assume that a given task either constitutes,
or does not constitute, a problem for all solvers.
Also, note that according to our definition CPS is not simply
an extension of "simple problem solving (henceforth
SPS), that is, problem solving involving relatively simple laboratory
problems. CPS and SPS are qualitatively different. For example,
whereas in SPS typically a single barrier needs to be overcome,
in CPS a large number of barriers exists. Because there are multiple
barriers, a single cognitive or behavioral activity may not be
sufficient to reach the goal state. Rather, a well-planned, prioritized,
set of cognitions and actions needs to be performed in order to
get closer to the goal state.
In addition, note that in contrast to earlier, often implicit
views, CPS is not viewed as deterministic in the sense that any
problem solving activity will always lead to the solution of a
problem. Rather, CPS may lead to an approximate solution that
may advance the solver but may not lead to actually solving the
problem. For example, subjects performing the duties of the mayor
of a computer-simulated town, may, even after some practice, still
not be able to generate the best possible solution to a given
problem. In fact, many, often computerized, tasks exist for which
-due to the complex nonlinear relations among the task variables-
the optimal solution is unknown. Of course, the absence of an
optimal solution, while theoretically reasonable and even desirable,
poses a problem to experimenters who want to determine the quality
of subjects' performances, and to those who use microworlds for
personnel selection purposes (e.g., U. Funke, 1995).
Finally, because both the given state and goal state and also
the barriers are intransparent in CPS, it is difficult for a solver
to evaluate her progress toward problem solution. This makes
it necessary for the solver to select and structure the interactions
with the task such that information that is helpful for the evaluation
of progress can be extracted.
A Theoretical Framework
for Complex Problem Solving
Adopting any definition has consequences not
only for how an area is studied but also for how empirical findings
are theoretically interpreted. For example, if problem solving
is defined in terms of cognitive, rather than neurophysiological,
biological, or behavioral, processes, then it makes little sense
to construct a theory of problem solving at a neurophysiological,
biological, or behavioral level. In the following, we present
our thoughts on how a general theoretical framework for understanding
problem solving that is based on our definition of CPS might look
like. Our framework is based on the assumptions that (a) the
theoretical goal of CPS research is to understand the interplay
among cognitive, motivational, personal, and social factors when
complex, novel, dynamic, intransparent tasks are solved, and (b)
the interplay among the various components can best be understood
within an Information Processing model. The framework is constrained,
of course, by what is known already about CPS as it is defined
above. Below, we therefore present a brief, non-exhaustive list
of the main empirical phenomena that have been demonstrated in
recent years, thereby summarizing many of the findings presented
in the various chapters of Frensch and Funke (1995).
Internal Subject Factors
(1) Experience. CPS appears to vary
with the amount of experience an individual has in the task domain
at hand (e.g., Krems, 1995). Experience affects the likelihood
of successful problem solving, but more importantly, it affects
which strategies are employed. It influences, for instance, whether
or not a person experiments with a task, that is, whether or not
the person exhaustively tests hypotheses about task relations
and tries to falsify the assumptions.
(2) Cognitive Variables. There is considerable evidence
that cognitive variables, such as background knowledge, monitoring
and evaluation strategies, and cognitive style affect CPS. There
is even evidence indicating that general intelligence, when measured
appropriately, affects at least some aspects of CPS (e.g., Beckmann
& Guthke, 1995). Also, it appears that at least under certain
conditions, CPS performance and explicit task knowledge may be
dissociable. That is, performance improvements can be found even
in the absence of explicit knowledge about the task (e.g., Berry
& Broadbent, 1995), although the interpretation of these dissociations
is not clear (see Buchner, Funke, & Berry, 1995).
(3) Non-cognitive Variables. CPS appears to be enhanced
by some non-cognitive factors such as self-confidence, perseverance,
motivation, and enjoyment. In general, both personality and social
factors appear to influence CPS (e.g., Dörner & Wearing,
1995). All these factors are included in theories of action regulation
which explain behavior as result of a complex interaction between
different modules. The modules deal not only with cognitive processes
but also with emotional states. Thus, the type of information
processing is influenced by non-cognitive variables.
External Factors
(1) Problem Structure. CPS appears
to vary with the structure of the task including the semantics
of the task, the complexity of the task, the transparency of the
task, and with other structural task features (e.g., J. Funke,
1995). Such structural variables appear to set up a frame within
which the problem solver makes certain "moves" that
depend on formal task characteristics.
(2) Problem Context. The likelihood of successful CPS
performance seems to vary with the semantic embeddedness of a
task, that is, with whether or not the task is couched within
a well understood and familiar context (e.g., Huber, 1995). In
a way, this external factor reflects the influence of the internal
factor experience mentioned above. The important role of contextual
embedding, known fairly well from deduction tasks and from cognitive
illusions, is also found in CPS.
(3) Environmental Factors. Successful CPS performance
is influenced by the environment within which a solver operates.
This includes feedback and feedback delay, expectations, cooperation,
peer pressure, and so on (e.g., Brehmer, 1995). Even if the task
at hand is kept constant, changes in the environment like a shift
from individual to group problem solving or from direct to delayed
feedback shows drastic effects on problem solving performance.
The Components of a Theory of CPS
These empirical findings have led
us to construct a simple theoretical framework for understanding
CPS that is depicted in Figure 1. The figure summarizes the basic
components of our framework and the interrelations among the components.
As can be seen, the framework contains three separate components,
the problem solver, the task, and the environment.
____________________
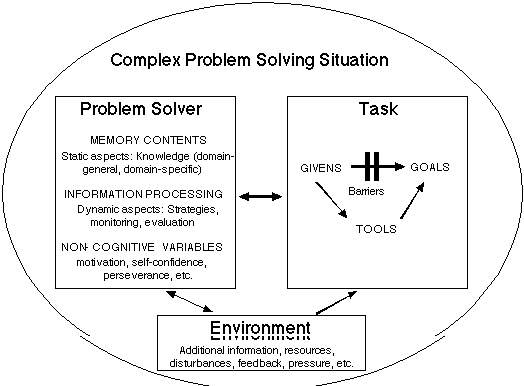
Figure 1.
CPS is viewed as the interaction between a problem solver and
a task in the context of an environment. The figure shows only
static aspects of the interaction. For additional information
see text.
____________________
Within the problem solver, we distinguish
between static memory content and dynamic information processing.
Memory is divided further into domain-general and domain-specific
knowledge both of which affect CPS performance. Information processing
includes the task strategies that are selected and the processes
of task monitoring and progress evaluation. In addition, non-cognitive
problem solver variables such as motivation and personality also
factor into CPS performance.
The task itself is depicted in terms of the barriers that exist
between a given state and a goal state (see our remarks on the
"gap definition). As noted above, the barriers are
assumed to be complex, dynamically changing, and intransparent;
the transition from given to goal state is constrained by the
problem solver's memory content and information processing, and
by the tools that are available to the solver.
The environment includes the resources that are available for
problem solving, as well as feedback, expectations, cooperation,
peer pressure, disturbances etc. The environment affects both
the problem solver and the task. It affects the problem solver
by constraining the information processes that can be used and
by influencing which knowledge is accessible. The environment
affects the task by offering additional information, constraining
which tools may be used, and so on. In addition, the environment
can be changed actively by the problem solver but not by the task.
From this relatively simple view of CPS, it
should become clear that two of the main questions that will need
to be addressed by future research are: (a) which components within
the problem solver, task, and environment affect CPS in which
way, and (b) how do the various components, the person, task,
and environment interact in affecting CPS performance? Clearly,
much more research will need to be conducted before we can attempt
to answer these questions.
Methodological Approaches
to Studying Complex Problem Solving
Of the many different methods that could potentially
be used to study CPS, two approaches have become favorites in
recent years, the experimental method and single-case studies
(cf. Eyferth, Schömann, & Widowski, 1986; Kluwe, 1993).
Although some researchers have argued that complex problem solving
cannot be fruitfully approached with classical experimental techniques
(e.g., Brehmer & Dörner, 1993; Dörner, 1992; Dörner
& Wearing, 1995; Schaub, 1993; Strohschneider, 1991), others
have demonstrated rather convincingly that experimental techniques
can be used to further our understanding of CPS (e.g., Funke,
1991, 1993; Hussy, 1985; Kluwe, 1995; Müller, 1993; Putz-Osterloh,
1993; Strauß, 1993). It now appears that the controversy
between supporters and opponents of the use of experimental methodology
may, at least in part, be based on a false conception of what
experimental methods really are. The experimental method is a
set of techniques, at least in our view, that can be employed
not only to test a set of static assumptions, but also to test
dynamic process models. Proponents of the experimentally oriented
research strategy argue that false theoretical models can be identified
only with the experimental method, that is, only experiments allow
one to make ultimate decisions about scientific hypotheses. Thus,
the experimental method may be most fruitfully employed for the
purpose of theory testing.
The phenomenologically oriented research strategy, on the other
hand, relies strongly on the precise reconstruction of single
cases of complex problem solving. Cognitive modeling is then
used to reconstruct certain aspects of an individual's behavior.
The procedure is described in detail by Kluwe (1995), who also
highlights some of the problematic features of this methodological
approach. In general, it appears to be rather difficult with
this approach to identify false theoretical assumptions. Proponents
of the single case methodology therefore frequently argue that
single cases are useful primarily for explorative purposes, i.e.,
for the development of scientific hypotheses.
We consider the two approaches, the experimental method and single
case studies, to be complementary. Single case studies may be
employed most fruitfully during theory development; the strength
of the experimental method in contrast, is that it provides a
strong test of proposed assumptions. While both of these approaches
are helpful and both have the potential to advance our understanding
of CPS, the effectiveness of the two approaches is critically
affected by some general problems of the research domain.
Addressing these problems is of critical importance before both
the experimental method and single case studies can be employed.
We list four of these general problems below:
(1) The first general problem concerns the
measurement of CPS knowledge and performance. The adequate
measurement of subjects' knowledge and performance in CPS situations
represents a major hurdle that needs to be addressed and resolved
before we can make any real progress toward understanding CPS.
To this end, Hübner (1989), for instance, has proposed mathematical
procedures for the operationalization of certain aspects of task
performance. Kolb, Petzing, and Stumpf (1992) propose the use
of operations research methods for the same purpose. We believe
that real progress will not come from these propositions but will
only come from theoretical advances. Any good theory of CPS must
prescribe the dependent variables and must outline how these variables
can be measured. Additionally, a theory of the formal system
itself may help to select important and reliable indicators of
system performance.
(2) The second general problem concerns generalizability and
external validity. Although the artificial systems currently
used in our labs are much more complex than they were 20 years
ago, we cannot necessarily assume that increased complexity has
also led to improved generalizability. Dörner's attempt
to bring complexity into the labs of the scholars of thinking
and problem solving was successful--but has the situation really
changed with respect to our understanding of real-world phenomena?
We agree with Hunt (1991, p. 391) who argues that "Geneticists
have a theory that explains how one generalizes from inheritance
in the fruit fly to inheritance in human beings. Cognitive psychology
does not have a theory to explain how we move from game behaviors
to behaviors in other situations.
(3) The third general problem concerns the analysis of problem
solving processes. It is of critical importance that we try
to understand the process of complex problem solving, rather than
the product. The experimental method has not been specifically
designed for process analyses, although experimental treatments
can help in testing assumptions about parameters and their assumed
dependence on external factors (e.g., multinomial modeling; see
Riefer & Batchelder, 1988). Thus, process models and experiments
are not contradictory; they are complementary tools that help
us understand CPS.
(4) And finally, the development of problem solving theories is
in a rather desolate condition. Developing a theory, or multiple
theories, is the most difficult job to achieve--and yet at the
same time the most necessary prerequisite for additional experimental
research. A good theory prescribes and determines experimental
research. Theoretical assumptions can be derived from everyday
experiences, from cognitive modeling, or from single-case or field
studies. Most, if not all, of the assumptions can be tested experimentally--but
neither the experimental method nor the single case approach prescribe
the development of theories.
It is our view that the experimental method
will remain one central method of choice for studying human CPS
simply because no other method is as capable of providing decisive
answers to clearly formulated questions. At the same time, however,
it remains clear that progress in this difficult research area
can be achieved only if different approaches work together to
achieve insights into how people deal with complex problems.
In conclusion, we believe that research on CPS, despite its many
shortcomings and despite the diverse approaches taken by North
American and European researchers, carries the promise of a more
realistic, real-life approach to the psychology of action control
within complex environments than has been employed in the past.
This is an exciting area of research, and as the old saying goes,
"Nothing is as promising as a beginning."
REFERENCES
Amsel, E., Langer, R., & Loutzenhiser,
L. (1991). Do lawyers reason differently from psychologists?
A comparative design for studying expertise. In R. J. Sternberg
& P. A. Frensch (Eds.), Complex problem solving: Principles
and mechanisms (pp. 223-250). Hillsdale, NJ: Lawrence Erlbaum
Associates.
Anderson, J. R. (1980). Cognitive psychology and its implications.
New York: W. H. Freeman and Company.
Anderson, J. R., Boyle, C. B., & Reiser, B. J. (1985). Intelligent
tutoring systems. Science, 228, 456-462.
Anzai, K., & Simon, H. A. (1979). The theory of learning
by doing. Psychological Review, 86, 124-140.
Beckmann, J. F., & Guthke, J. (1995). Complex problem solving,
intelligence, and learning ability. In P. A. Frensch & J. Funke (Eds.), Complex problem solving: The European Perspective (pp. 177-200). Hillsdale, NJ: Lawrence Erlbaum Associates.
Berry, D. C., & Broadbent, D. E. (1995). Implicit learning
in the control of complex systems: A reconsideration of some of
the earlier claims. In P.A. Frensch & J. Funke (Eds.), Complex
problem solving: The European Perspective (pp. 131-150).
Hillsdale, NJ: Lawrence Erlbaum Associates.
Bhaskar, R., & Simon, H. A. (1977). Problem solving in semantically
rich domains: An example from engineering thermodynamics. Cognitive
Science, 1, 193-215.
Brehmer, B. (1995). Feedback delays in dynamic decision making.
In P. A. Frensch & J. Funke (Eds.), Complex problem solving:
The European Perspective (pp. 103-130). Hillsdale, NJ: Lawrence
Erlbaum Associates.
Brehmer, B., & Dörner, D. (1993). Experiments with
computer-simulated microworlds: Escaping both the narrow straits
of the laboratory and the deep blue sea of the field study. Computers
in Human Behavior, 9, 171-184.
Broadbent, D. E. (1977). Levels, hierarchies, and the locus
of control. Quarterly Journal of Experimental Psychology,
29, 181-201.
Bryson, M., Bereiter, C., Scardamalia, M., & Joram, E. (1991).
Going beyond the problem as given: Problem solving in expert
and novice writers. In R. J. Sternberg & P. A. Frensch (Eds.),
Complex problem solving: Principles and mechanisms (pp.
61-84). Hillsdale, NJ: Lawrence Erlbaum Associates.
Buchner, A. (1995). Theories of complex problem solving. In
P. A. Frensch & J. Funke (Eds.), Complex problem solving:
The European Perspective (pp. 27-63). Hillsdale, NJ: Lawrence
Erlbaum Associates.
Buchner, A., Funke, J., & Berry, D. C. (1995). Negative correlations
between control performance and verbalizable knowledge: Indicators
for implicit learning in process control tasks? Quarterly
Journal of Experimental Psychology, 48A, 166-187.
Chase, W. G., & Simon, H. A. (1973). Perception in chess.
Cognitive Psychology, 4, 55-81.
Chi, M. T. H., Feltovich, P. J., & Glaser, R. (1981). Categorization
and representation of physics problems by experts and novices.
Cognitive Science, 5, 121-152.
Dörner, D. (1975). Wie Menschen eine Welt verbessern wollten
[How people wanted to improve the world]. Bild der Wissenschaft,
12, 48-53.
Dörner, D. (1985). Verhalten, Denken und Emotionen [Behavior,
thinking, and emotions]. In L. H. Eckensberger & E. D. Lantermann
(Eds.), Emotion und Reflexivität (pp. 157-181). München,
Germany: Urban & Schwarzenberg.
Dörner, D. (1992). Über die Philosophie der Verwendung
von Mikrowelten oder "Computerszenarios" in der psychologischen
Forschung [On the proper use of microworlds or "computer
scenarios" in psychological research]. In H. Gundlach (Ed.),
Psychologische Forschung und Methode: Das Versprechen des Experiments.
Festschrift für Werner Traxel (pp. 53-87). Passau, Germany:
Passavia-Universitäts-Verlag.
Dörner, D., Kreuzig, H. W., Reither, F., & Stäudel,
T. (Eds.). (1983). Lohhausen. Vom Umgang mit Unbestimmtheit
und Komplexität [Lohhausen. On dealing with uncertainty
and complexity]. Bern, Switzerland: Hans Huber.
Dörner, D., & Wearing, A. (1995). Complex problem solving:
Toward a (computer-simulated) theory. In P. A. Frensch & J. Funke (Eds.), Complex problem solving: The European Perspective (pp. 65-99). Hillsdale, NJ: Lawrence Erlbaum Associates.
Duncker, K. (1935). Zur Psychologie des produktiven Denkens
[The psychology of productive thinking]. Berlin: Julius Springer.
Ewert, P. H., & Lambert, J. F. (1932). Part II: The effect
of verbal instructions upon the formation of a concept. Journal
of General Psychology, 6, 400-411.
Eyferth, K., Schömann, M., & Widowski, D. (1986). Der
Umgang von Psychologen mit Komplexität [On how psychologists
deal with complexity]. Sprache & Kognition, 5,
11-26.
Frensch, P. A., & Funke, J. (Eds.). (1995). Complex problem
solving: The European Perspective. Hillsdale, NJ: Lawrence
Erlbaum Associates.
Frensch, P. A., & Sternberg, R. J. (1991). Skill-related
differences in game playing. In R. J. Sternberg & P. A. Frensch
(Eds.), Complex problem solving: Principles and mechanisms
(pp. 343-381). Hillsdale, NJ: Lawrence Erlbaum Associates.
Funke, J. (1991). Solving complex problems: Human identification
and control of complex systems. In R. J. Sternberg & P. A.
Frensch (Eds.), Complex problem solving: Principles and mechanisms
(pp. 185-222). Hillsdale, NJ: Lawrence Erlbaum Associates.
Funke, J. (1993). Microworlds based on linear equation systems:
A new approach to complex problem solving and experimental results.
In G. Strube & K.-F. Wender (Eds.), The cognitive psychology
of knowledge (pp. 313-330). Amsterdam: Elsevier Science Publishers.
Funke, J. (1995). Experimental research on complex problem solving.
In P. A. Frensch & J. Funke (Eds.), Complex problem solving:
The European Perspective (pp. 243-268). Hillsdale, NJ: Lawrence
Erlbaum Associates.
Funke, U. (1995). Complex problem solving in personnel selection
and training. In P. A. Frensch & J. Funke (Eds.), Complex
problem solving: The European Perspective (pp. 219-240).
Hillsdale, NJ: Lawrence Erlbaum Associates.
Groner, M., Groner, R., & Bischof, W. F. (1983). Approaches
to heuristics: A historical review. In R. Groner, M. Groner,
& W. F. Bischof (Eds.), Methods of heuristics (pp.
1-18). Hillsdale, NJ: Lawrence Erlbaum Associates.
Hayes, J. (1980). The complete problem solver. Philadelphia:
The Franklin Institute Press.
Hegarty, M. (1991). Knowledge and processes in mechanical problem
solving. In R. J. Sternberg & P. A. Frensch (Eds.), Complex
problem solving: Principles and mechanisms (pp. 253-285).
Hillsdale, NJ: Lawrence Erlbaum Associates.
Heppner, P. P., & Krauskopf, C. J. (1987). An information-processing
approach to personal problem solving. The Counseling Psychologist,
15, 371-447.
Huber, O. (1995). Complex problem solving as multi stage decision
making. In P. A. Frensch & J. Funke (Eds.), Complex problem
solving: The European Perspective (pp. 151-173). Hillsdale,
NJ: Lawrence Erlbaum Associates.
Hübner, R. (1989). Methoden zur Analyse und Konstruktion
von Aufgaben zur kognitiven Steuerung dynamischer Systeme [Methods
for the analysis and construction of dynamic system control tasks].
Zeitschrift für Experimentelle und Angewandte Psychologie,
36, 221-238.
Hunt, E. (1991). Some comments on the study of complexity.
In R. J. Sternberg, & P. A. Frensch (Eds.), Complex problem
solving: Principles and mechanisms (pp. 383-395). Hillsdale,
NJ: Lawrence Erlbaum Associates.
Hussy, W. (1985). Komplexes Problemlösen - Eine Sackgasse?
[Complex problem solving - a dead end?]. Zeitschrift für
Experimentelle und Angewandte Psychologie, 32, 55-77.
Kay, D. S. (1991). Computer interaction: Debugging the problems.
In R. J. Sternberg & P. A. Frensch (Eds.), Complex problem
solving: Principles and mechanisms (pp. 317-340). Hillsdale,
NJ: Lawrence Erlbaum Associates.
Kluwe, R. H. (1993). Knowledge and performance in complex problem
solving. In G. Strube & K.-F. Wender (Eds.), The cognitive
psychology of knowledge (pp. 401-423). Amsterdam: Elsevier
Science Publishers.
Kluwe, R. H. (1995). Single case studies and models of complex
problem solving. In #, Complex
problem solving: The European Perspective (pp. 269-291).
Hillsdale, NJ: Lawrence Erlbaum Associates.
Kolb, S., Petzing, F., & Stumpf, S. (1992). Komplexes Problemlösen:
Bestimmung der Problemlösegüte von Probanden mittels
Verfahren des Operations Research - ein interdisziplinärer
Ansatz [Complex problem solving: determining the quality of human
problem solving by operations research tools - an interdisciplinary
approach]. Sprache & Kognition, 11, 115-128.
Krems, J. F. (1995). Cognitive flexibility and complex problem
solving. In P. A. Frensch & J. Funke (Eds.), Complex problem
solving: The European Perspective (pp. 201-218). Hillsdale,
NJ: Lawrence Erlbaum Associates.
Lesgold, A., & Lajoie, S. (1991). Complex problem solving
in electronics. In R. J. Sternberg & P. A. Frensch (Eds.),
Complex problem solving: Principles and mechanisms (pp.
287-316). Hillsdale, NJ: Lawrence Erlbaum Associates.
Mayer, R. E. (1992). Thinking, problem solving, cognition.
Second edition. New York: W. H. Freeman and Company.
Müller, H. (1993). Komplexes Problemlösen: Reliabilität
und Wissen [Complex problem solving: Reliability and knowledge].
Bonn, Germany: Holos.
Newell, A., & Simon, H. A. (1972). Human problem solving.
Englewood Cliffs, NJ: Prentice-Hall.
Putz-Osterloh, W. (1993). Strategies for knowledge acquisition
and transfer of knowledge in dynamic tasks. In G. Strube &
K.-F. Wender (Eds.), The cognitive psychology of knowledge
(pp. 331-350). Amsterdam: Elsevier Science Publishers.
Riefer, D.M., & Batchelder, W.H. (1988). Multinomial modeling
and the measurement of cognitive processes. Psychological
Review, 95, 318-339.
Ringelband, O. J., Misiak, C., & Kluwe, R. H. (1990). Mental
models and strategies in the control of a complex system. In
D. Ackermann, & M. J. Tauber (Eds.), Mental models and
human-computer interaction (Vol. 1, pp. 151-164). Amsterdam:
Elsevier Science Publishers.
Schaub, H. (1993). Modellierung der Handlungsorganisation.
Bern, Switzerland: Hans Huber.
Sokol, S. M., & McCloskey, M. (1991). Cognitive mechanisms
in calculation. In R. J. Sternberg & P. A. Frensch (Eds.),
Complex problem solving: Principles and mechanisms (pp.
85-116). Hillsdale, NJ: Lawrence Erlbaum Associates.
Stanovich, K. E., & Cunningham, A. E. (1991). Reading as
constrained reasoning. In R. J. Sternberg & P. A. Frensch
(Eds.), Complex problem solving: Principles and mechanisms
(pp. 3-60). Hillsdale, NJ: Lawrence Erlbaum Associates.
Sternberg, R. J. (1995). Conceptions of expertise in complex
problem solving: A comparison of alternative conceptions. In
P. A. Frensch & J. Funke (Eds.), Complex problem solving:
The European Perspective (pp. 295-321). Hillsdale, NJ: Lawrence
Erlbaum Associates.
Sternberg, R. J., & Frensch, P. A. (Eds.). (1991). Complex
problem solving: Principles and mechanisms. Hillsdale, NJ:
Lawrence Erlbaum Associates.
Strauß, B. (1993). Konfundierungen beim Komplexen Problemlösen.
Zum Einfluß des Anteils der richtigen Lösungen (ArL)
auf das Problemlöseverhalten in komplexen Situationen
[Confoundations in complex problem solving. On the influence of
the degree of correct solutions on problem solving in complex
situations]. Bonn, Germany: Holos.
Strohschneider, S. (1991). Kein System von Systemen! Kommentar
zu dem Aufsatz "Systemmerkmale als Determinanten des Umgangs
mit dynamischen Systemen" von Joachim Funke [No system of
systems! Reply to the paper "System features as determinants
of behavior in dynamic task environments" by Joachim Funke].
Sprache & Kognition, 10, 109-113.
Van Lehn, K. (1989). Problem solving and cognitive skill acquisition.
In M. I. Posner (Ed.), Foundations of cognitive science
(pp. 527-579). Cambridge, MA: MIT Press.
Voss, J. F., Wolfe, C. R., Lawrence, J. A., & Engle, R. A.
(1991). From representation to decision: An analysis of problem
solving in international relations. In R. J. Sternberg &
P. A. Frensch (Eds.), Complex problem solving: Principles and
mechanisms (pp. 119-158). Hillsdale, NJ: Lawrence Erlbaum
Associates.
Wagner, R. K. (1991). Managerial problem solving. In R. J.
Sternberg & P. A. Frensch (Eds.), Complex problem solving:
Principles and mechanisms (pp. 159-183). Hillsdale, NJ: Lawrence
Erlbaum Associates.
Authors' Note
The present manuscript is a condensed and
revised version of Chapter 1 from Frensch and Funke (1995).
A version of this paper appeared in Russian language under the title:
Funke, J. & Frensch, P. A. (1995). Complex problem solving research
in North America and Europe: An integrative review. Foreign Psychology, 5, 42-47.
Send correspondence to Joachim Funke, Psychologisches Institut der
Universität Heidelberg, Hauptstr. 47-51, D-69117 Heidelberg, Germany,
or to Peter A. Frensch, Humboldt-University, Institut für Psychologie, Hausvogteiplatz 5-7, D-10117 Berlin, Germany. Electronic
mail may be sent to: joachim.funke@psychologie.uni-heidelberg.de or
peter.frensch@psychologie.hu-berlin.de.
ABOUT THE AUTHORS
Joachim Funke was born in 1953, and studied psychology, philosophy, and German
at the Universities of Duesseldorf, Basel (Switzerland), and Trier.
He received his M.S. in psychology in 1980, and was awarded his
Ph.D. in 1984 at the University of Trier. In 1985, he moved to
the University of Bonn, where he was granted his habilitation
in 1990. From 1991 until 1997 he was Associate Professor at Bonn University.
He is now in the Department of Psychology
at Heidelberg University as Full Professor. His research interests include
all areas of Experimental Psychology, with a special interest in cognitive
processes in memory and problem solving.
Peter A. Frensch was born in 1956, and studied electrical
engineering, psychology, and philosophy at the Universities of
Darmstadt and Trier, Germany, and at Yale University, USA. He
received his M.S. in 1987, followed by his M.phil. in 1988, and
his Ph.D. in 1989, and has been working as an Assistant Professor
of Psychology in the Department of Psychology at the University
of Missouri-Columbia, USA, from 1989 until 1995. After working for some years at the Max-Planck-Institute for Human Development and Education, he is now Full Professor at the Department of Psychology, Humboldt-University, Berlin, Germany. His research interests include learning, memory,
and problem solving.
|
|